Introduction
Understanding statistical data types is fundamental to making sense of the vast amounts of information generated in fields like healthcare, economics, and social sciences.
To truly understand this discipline, we must first comprehend the statistical data types and how we obtain them.
Type of Data
This question lies at the very foundation of statistical understanding, as it shapes how we approach data analysis and interpretation. In essence, data serves as our window into reality, providing numerical representations of real-world phenomena that we can systematically analyze. These representations are acquired through two primary methods: counting and measuring. From these procedures, we obtain two main data types. When we obtain data through counting processes, we create what statisticians refer to as “discrete” variables – these are distinct, separate values with clear boundaries between them. On the other hand, when we gather data through measurement processes, we work with “continuous” variables, which can theoretically take any value within their defined range.
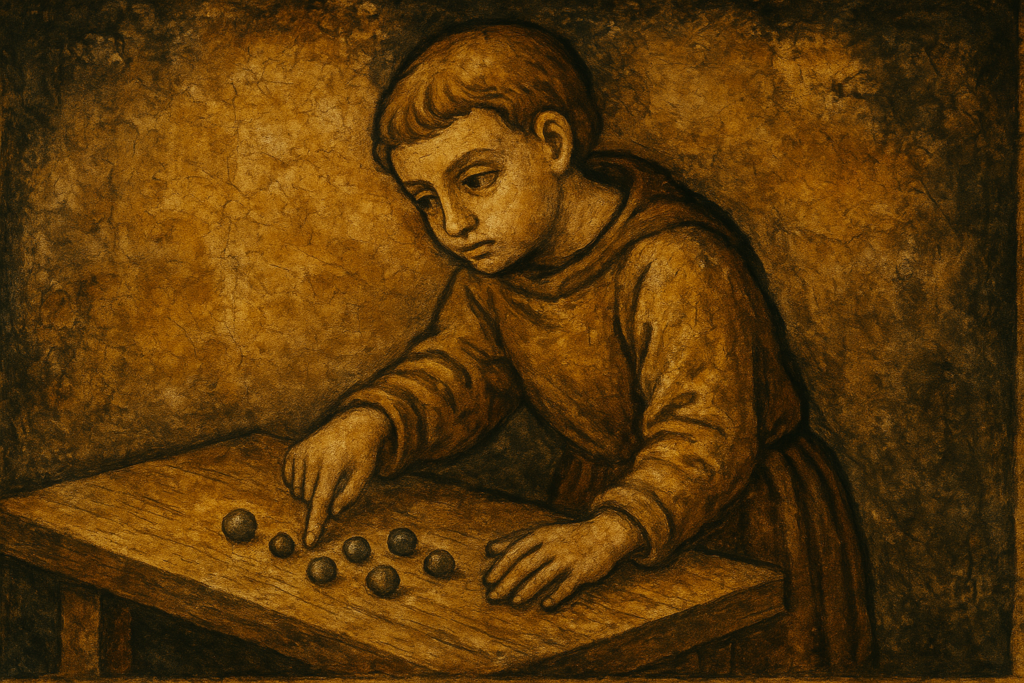
Discrete variables
Discrete variables are characterized by their ability to take only specific, countable values, which are typically represented as integers with clear separations between possible values.
In statistical analysis, these variables are fundamental because they represent phenomena that can be counted rather than measured continuously.
For instance, when counting objects or occurrences, we encounter discrete variables such as the number of candies contained within a bag, the total count of patients diagnosed with a particular disease in a hospital ward, the size of families measured by the number of children, or the precise quantity of tablets prescribed in a medical prescription.
These types of variables have distinct gaps between their possible values – there cannot be 2.5 children in a family or 3.7 tablets in a prescription.
Because of their clear-cut nature, discrete variables are particularly well-suited to representation through structured formats such as tables or visual displays like bar charts, which effectively communicate the frequency or distribution of these distinct values and facilitate comprehensive analysis and interpretation of the data.
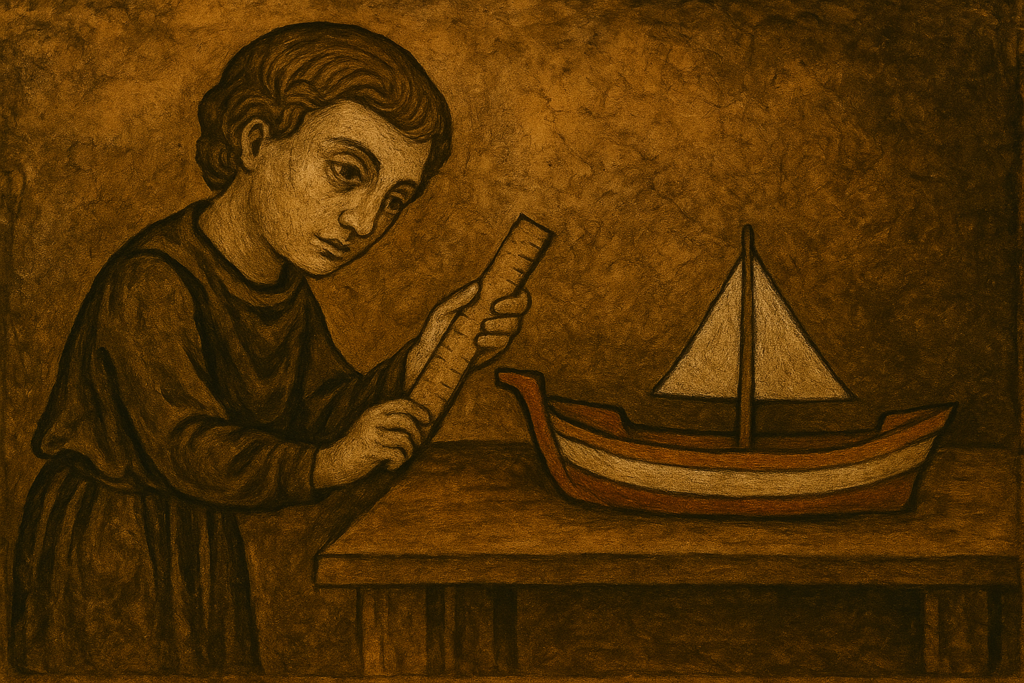
Continuous variables
Continuous variables represent measurements that can assume any numerical value within a specified range, including precise decimal and fractional quantities. Unlike discrete variables that have clear gaps between values, continuous variables flow smoothly from one value to another without interruption.
In scientific and medical contexts, we frequently encounter continuous variables in measurements such as body weight (which can be recorded with increasing precision down to fractions of grams), blood pressure readings (which can fluctuate by minute amounts), and body temperature (which can be measured to multiple decimal places).
The inherent nature of continuous variables makes them particularly well-suited for visualization through smooth curves and line graphs, which effectively illustrate how these measurements vary across their full range. When plotting continuous data, we often observe natural patterns or distributions that reveal important trends and relationships, providing valuable insights into the underlying phenomena being studied.
Categorical variables
This definition of “data” requires expansion because it primarily focuses on numerical, quantitative data, which represents only one aspect of the broader data landscape. In statistical analysis, we must also consider non-numerical, qualitative data, which carry equal importance and significance. These qualitative data elements, while not expressed in numerical terms, encapsulate crucial categorical information that provides essential context and classification. Common examples in medical and demographic studies include blood group classifications (A, B, AB, O), gender identifications, disease staging categories, and other descriptive characteristics. Such qualitative data elements, though not mathematically manipulatable in the same way as quantitative data, form an integral part of comprehensive statistical analysis, enabling researchers to identify patterns, establish relationships, and draw meaningful conclusions about populations and phenomena under study. These types of data provide valuable insights that complement quantitative measurements, creating a more complete and nuanced understanding of the subject matter being investigated.
Ordinal variable
Additionally, there exists an important category known as ordinal data that occupies a unique position between qualitative and quantitative data type. This classification is particularly interesting because it combines elements of both categories in a structured way. For instance, a pain scale ranging from 1 to 10 is a classic example of ordinal data in medical settings.
Similarly, customer satisfaction ratings (very dissatisfied to very satisfied) and educational achievement levels (elementary, secondary, undergraduate, graduate) are common instances of ordinal data in social research.
While these data types are organized in a meaningful hierarchical sequence that allows for clear ranking, they possess a distinctive characteristic: consecutive ranks do not have uniform or equal intervals or distances between them
This means that while we can definitively say that a pain level of 8 indicates more severe pain than a level of 4, we cannot conclude that the difference in pain intensity between levels 8 and 6 is equivalent to the difference between levels 6 and 4.
Calculated and Derived Data
Not all data comes from primary methods of counting or measuring. Analysts and researchers often derive or calculate data from other existing data points using mathematical operations or established formulas.
Healthcare professionals, for example, calculate a patient’s BMI (Body Mass Index) by using the relationship between weight and height measurements. Similarly, researchers derive metabolic rate calculations, population density figures, and economic indicators like GDP per capita from multiple primary data points.
Analysts can transform quantitative data into various formats – they might express it in binary form (such as yes/no, present/absent), convert it into categorical rankings, or encode it using specialized systems that move beyond traditional numerical representations. These derived and encoded forms of data serve a crucial role in advanced statistical analysis and data interpretation, showing how we can transform raw data into more meaningful and applicable formats.
Conclusion
Understanding the nature and different data type is fundamental for any effective statistical analysis. We have explored how data can manifest in various forms: discrete for precise counts, continuous for measurements, categorical for qualitative classifications, and ordinal for hierarchical scales. Additionally, we have seen how some data can be derived from others through calculations and formulas.
This comprehensive understanding of data types is essential for:
Choosing the most appropriate analysis methods
Correctly interpreting statistical result
Effectively representing information
Making informed data-based decision
In an increasingly data-driven era, this fundamental knowledge becomes an indispensable tool for anyone involved in statistical analysis, research, or data-based decision-making.